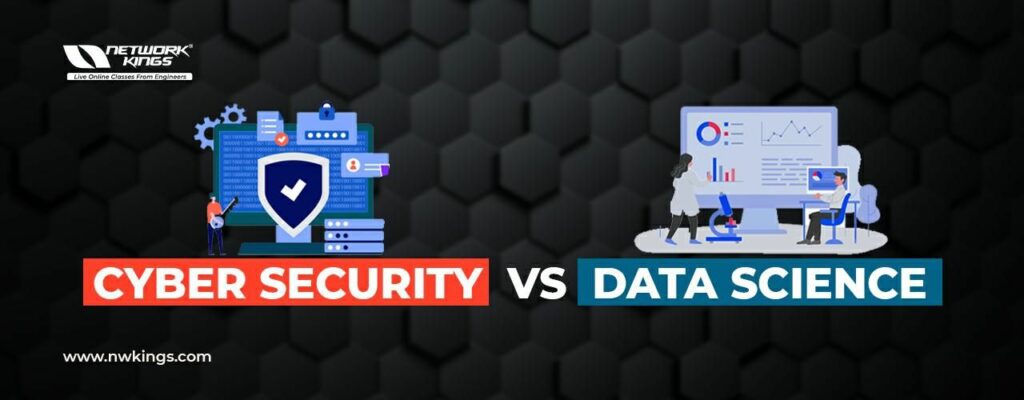
Do you want to know the difference between cyber security and data science? Cyber Security vs Data Science has become a crucial topic these days. So, if you too, want to learn about the two domains of IT, keep reading the blog till the end.
What is Cyber Security?
Cybersecurity is a broad field focused on shielding computer systems, networks, and data from unauthorized access, attacks, or theft. In our increasingly digital world, where information holds immense value, cybersecurity becomes crucial for safeguarding individuals, organizations, and governments.
The main objective of cybersecurity is to ensure that information remains confidential, intact, and accessible. This involves implementing various technical measures, processes, and policies to counter a wide array of cyber threats like malware, phishing, ransomware, and hacking. Security measures include tools like firewalls, encryption, multi-factor authentication, and intrusion detection systems.
Professionals in cybersecurity constantly adapt to emerging threats, employing proactive strategies to identify vulnerabilities and manage risks. Ethical hacking, security audits, and ongoing education play vital roles in maintaining a robust cybersecurity framework. Collaboration among government bodies, private businesses, and individuals is essential to form a collective defence against cyber threats. With technological advancements, the significance of cybersecurity continues to grow, establishing it as a fundamental aspect of our interconnected and digital society.
What is the importance of Cyber Security?
Cybersecurity holds immense importance in our interconnected digital landscape. It involves a set of practices, technologies, and measures crafted to shield computer systems, networks, and data from unauthorized access, attacks, and harm. The importance of cyber security can be understood as follows-
- Safeguarding Sensitive Information
In our digital era, businesses, governments, and individuals amass extensive sensitive information. This encompasses personal data, financial records, intellectual property, and confidential business details. Cybersecurity serves as a protective barrier, thwarting theft, unauthorized access, and misuse of this critical information.
- Mitigating Financial Loss
Cyberattacks pose a real threat of substantial financial losses to both organizations and individuals. Cybersecurity measures act as a defence, preventing financial theft, fraud, and disruptions to financial systems.
- Preserving Personal Privacy
Respecting personal privacy is a fundamental right, and cybersecurity plays a pivotal role in upholding it. Through various measures, cybersecurity safeguards individuals’ personal information from exploitation and misuse by unauthorized entities.
- Ensuring Business Continuity
Cyberattacks can disrupt normal business operations, resulting in downtime, decreased productivity, and financial setbacks. Robust cybersecurity practices are a cornerstone for business continuity, averting and mitigating the impact of cyber incidents.
- Securing Critical Infrastructure
Critical infrastructure, such as power grids, water supplies, transportation systems, and healthcare facilities, relies heavily on cybersecurity for protection. Attacks on these systems could have dire consequences for public safety and national security.
- Contributing to National Security
In the modern age, national security is intricately linked to cybersecurity. Governments and military organizations depend on secure communication channels, data protection, and defence against cyber threats to uphold the integrity of their operations.
- Combating Cybercrime
Cybersecurity measures are indispensable in the fight against cybercrime, encompassing hacking, identity theft, ransomware, and other malicious activities. Effective cybersecurity aids law enforcement agencies in tracking, apprehending, and prosecuting cybercriminals.
- Building Trust and Reputation
Organizations that give priority to cybersecurity cultivate trust with their customers, partners, and stakeholders. A breach in security can tarnish a company’s reputation, erode trust, and lead to lasting consequences.
- Addressing Global Interconnectedness
The internet’s interconnected nature means that a cyber incident in one part of the world can have far-reaching effects globally. Cybersecurity is pivotal in maintaining the stability and reliability of the digital ecosystem.
- Promoting Innovation and Technological Advancement
Cybersecurity is essential for fostering innovation and technological progress. As more aspects of our lives become digitized, a secure digital environment encourages the development and adoption of new technologies.
What is the scope of cybersecurity?
The scope of cybersecurity is broad and constantly evolving with advancements in technology and the increasing sophistication of digital threats. It can be explained as follows-
- Network Security
Safeguarding computer networks against unauthorized access, attacks, and vulnerabilities using measures like firewalls and encryption protocols.
- Information Security
Protecting sensitive data from unauthorized access, alteration, and destruction through strategies like access controls and encryption.
- Endpoint Security
Ensuring the security of individual devices such as computers, smartphones, and IoT devices with tools like antivirus software and endpoint protection.
- Application Security
Focusing on securing software applications, including web and mobile apps, to prevent vulnerabilities and unauthorized access.
- Cloud Security
Securing data, applications, and infrastructure in cloud computing environments using measures like identity management and encryption.
- Identity and Access Management (IAM)
Managing and controlling user access to systems and resources to ensure authorized individuals have appropriate access levels.
- Incident Response and Forensics
Developing plans and procedures to respond to cybersecurity incidents and conducting digital forensics to analyze security breaches.
- Security Operations
Monitoring and managing security events in real-time, including threat detection, incident response, and continuous system monitoring.
- Security Policy and Governance
Developing and implementing security policies, standards, and procedures for compliance with regulations and best practices.
- Security Awareness and Training
Educating users and employees about cybersecurity best practices and promoting a security-conscious culture within organizations.
- Critical Infrastructure Protection
Securing essential systems and services, such as power grids and transportation networks, to prevent cyber threats with severe consequences.
- Internet of Things (IoT) Security
Ensuring the security of connected devices and IoT ecosystems to prevent unauthorized access and exploitation of vulnerabilities.
- Cybersecurity Policy and Law
Developing and enforcing laws, regulations, and policies related to cybersecurity at national and international levels.
- Ethical Hacking and Penetration Testing
Conducting simulated attacks to identify vulnerabilities in systems and networks, helping organizations address security weaknesses.
- Security Research and Development
Advancing the field through research and developing new technologies, tools, and methodologies to stay ahead of emerging cyber threats.
What are the core concepts of Cyber Security?
The core concepts of cyber security are as follows-
- Confidentiality
Confidentiality means guaranteeing that only authorized individuals or entities can access sensitive information. It includes utilizing encryption, access controls, and secure communication protocols.
- Integrity
Integrity means preserving the accuracy and trustworthiness of data and information throughout its lifecycle. It includes employing data validation, checksums, and digital signatures.
- Availability
Availability refers to ensuring that systems and data remain accessible and functional without disruption when needed. It includes incorporating redundancy, backups, and robust disaster recovery plans.
- Authentication
Authentication refers to verifying the identity of users, systems, or devices to confirm their claimed identity. It includes employing methods such as passwords, biometrics, and multi-factor authentication.
- Authorization
Authorization refers to granting appropriate access privileges to authenticated users, restricting access to specific resources. It includes utilizing access controls and role-based access control (RBAC).
- Non-Repudiation
Non-repudiation refers to ensuring that a user cannot deny the authenticity of their actions or transactions. It includes employing digital signatures, transaction logs, and audit trails.
- Risk Management
Risk Management refers to identifying, assessing, and mitigating risks to an organization’s information assets. It includes conducting risk assessments, vulnerability assessments, and implementing risk mitigation strategies.
- Security Policies
Security Policies refer to documented guidelines and rules that articulate the organization’s approach to cybersecurity. It includes establishing acceptable use policies, data protection policies, and incident response plans.
- Incident Response
Incident Response refers to a structured approach to addressing and managing the aftermath of a cybersecurity incident. It includes developing incident response plans, and communication protocols, and conducting forensic analysis.
- Security Awareness and Training
Security Awareness and Training refers to educating users and employees on cybersecurity best practices and fostering a security-conscious culture. It includes implementing training programs, and awareness campaigns, and providing regular security updates.
- Cryptography
Cryptography refers to utilizing mathematical techniques to secure communication and protect information. It includes employing encryption algorithms, digital signatures, and secure key management.
- Firewalls and Network Security
Firewall and Network Security refers to establishing barriers to control and monitor incoming and outgoing network traffic. It includes utilizing firewalls, intrusion detection/prevention systems, and designing secure network architectures.
- Security Architecture
Security Architecture refers to designing and implementing a secure structure for systems and networks. It includes creating a secure network design, implementing layered security, and adopting a defence-in-depth approach.
- Security Governance
Security Governance refers to establishing and maintaining a framework for managing and overseeing an organization’s cybersecurity efforts. It includes demonstrating leadership commitment, defining policies, and providing oversight for the security program.
- Vulnerability Management
Vulnerability Management refers to identifying, prioritizing, and mitigating vulnerabilities in systems and applications. It includes conducting regular vulnerability assessments, implementing patch management, and staying current with security updates.
What are the key technologies in Cyber Security?
The key technologies in cyber security are as follows-
- Firewalls
It monitors and regulates incoming and outgoing network traffic based on predefined security rules and includes hardware firewalls, software firewalls, and next-generation firewalls.
- Intrusion Detection Systems (IDS) and Intrusion Prevention Systems (IPS)
It identifies and responds to potential security threats or incidents and comprises network-based IDS/IPS and host-based IDS/IPS.
- Antivirus and Antimalware Software
It detects, prevents, and eliminates malicious software such as viruses, worms, and Trojans and is deployed on endpoints, servers, and network gateways.
- Virtual Private Networks (VPNs)
It establishes secure, encrypted connections over untrusted networks and encompasses remote-access VPNs and site-to-site VPNs.
- Encryption Technologies
It safeguards sensitive data by encoding it to be deciphered only by authorized parties and involves symmetric encryption, asymmetric encryption, SSL, and TLS.
- Multi-Factor Authentication (MFA)
It requires users to provide multiple forms of identification before granting access and utilizes biometrics, smart cards, and token-based authentication.
- Security Information and Event Management (SIEM)
It collects, analyzes, and correlates log data to detect and respond to security events and encompasses SIEM solutions and platforms.
- Endpoint Protection
It safeguards individual devices (endpoints) from security threats and involves antivirus software, endpoint detection and response (EDR), and mobile device management (MDM).
- Web Application Firewalls (WAF)
It shields web applications from various online threats and gets placed between web applications and the internet to filter and monitor HTTP traffic.
- Network Segmentation
It divides a network into segments to limit the spread of potential security breaches and incorporates firewalls and VLANs (Virtual Local Area Networks).
- Security Assessment and Penetration Testing Tools
It identifies and evaluates vulnerabilities in systems and networks and utilizes tools such as Nessus, Metasploit, and Burp Suite.
- Identity and Access Management (IAM)
It manages user identities and controls access to resources based on roles and permissions and encompasses Single Sign-On (SSO) and IAM platforms.
- Security Orchestration, Automation, and Response (SOAR)
It streamlines and automates incident response processes and involves SOAR platforms and solutions.
- Blockchain Technology
It provides a decentralized and secure method for recording and verifying transactions and is applied to secure digital transactions and ensure data integrity.
- Artificial Intelligence (AI) and Machine Learning (ML)
It enhances threat detection and response capabilities through pattern recognition and anomaly detection and involves ML algorithms for behaviour analysis and AI-driven threat intelligence.
- Container Security
It secures applications and their environments within containers and encompasses container security platforms and runtime security tools.
- Cloud Security
It ensures the security of data, applications, and infrastructure in cloud environments and involves Cloud Access Security Brokers (CASB), encryption, and identity management in the cloud.
- Biometric Authentication
It authenticates individuals based on unique physical or behavioural characteristics and includes fingerprint recognition, facial recognition, and iris scanning.
How to become a Cyber Security professional? - Cybersecurity Specialists
Embarking on a journey to become a cybersecurity professional involves a blend of education, hands-on experience, and a commitment to continuous learning to navigate the swiftly evolving landscape. Here is a step-by-step guide to forging a career in cybersecurity:
- Educational Foundation
Bachelor’s Degree: Attain a bachelor’s degree in a relevant field like computer science, information technology, cybersecurity, or a related discipline. This lays the groundwork for understanding computer systems and networks.
- Specialized Education and Certifications
Cybersecurity Certifications: Pursue industry-recognized certifications to validate your expertise. Key certifications include:
- CompTIA Security+
- CompTIA PenTest+
- Certified Information Systems Security Professional (CISSP)
- Certified Ethical Hacker (CEH)
- Certified Information Security Manager (CISM)
- Networking and Computer Proficiency
Cultivate robust networking and computer skills. Familiarize yourself with operating systems (Windows, Linux), networking protocols, and fundamental system administration tasks.
- Programming and Scripting
Acquire proficiency in programming languages commonly used in cybersecurity, such as Python, Java, or PowerShell. Programming skills are invaluable for tasks like automation, scripting, and comprehending malware.
- Practical Experience
Garner practical experience through internships, part-time roles, or entry-level positions. Engage in real-world projects to apply and enhance your knowledge and skills.
- Home Lab Setup
Establish a virtual lab environment to experiment with cybersecurity tools and techniques. This provides hands-on experience in a secure and controlled setting.
- Stay Informed
Keep abreast of industry news, blogs, and forums to stay current on the latest cybersecurity trends, threats, and technologies. Subscribe to cybersecurity publications and attend relevant conferences.
- Networking and Community Engagement
Engage with online forums, attend local meetups, and actively participate in cybersecurity communities. Networking with professionals in the field can offer valuable insights, mentorship, and job opportunities.
- Continuous Learning
Embrace the dynamic nature of cybersecurity by committing to continuous learning. Stay informed about emerging technologies, new threats, and evolving best practices.
- Specialization in a Niche Area
Recognize the various specializations within cybersecurity, such as penetration testing, incident response, and threat hunting. Identify an area that aligns with your interests and specialize in it.
- Soft Skills Development
Hone communication and problem-solving skills. Effective communication is crucial as cybersecurity professionals often need to convey complex technical issues to non-technical stakeholders.
- Entry-Level Job Applications
Apply for entry-level positions such as cybersecurity analyst, junior penetration tester, or security administrator to gain practical experience in a professional setting.
- Engage in Ethical Hacking and Bug Bounty Programs
Participate in ethical hacking and engage in bug bounty programs. This provides a legal avenue to practice your skills and potentially earn rewards for identifying vulnerabilities.
- Adherence to Ethical Standards
Uphold ethical standards in your work. Given the sensitive nature of information handled in cybersecurity, trust is paramount in the field.
Discuss the cybersecurity career path.
Navigating the cybersecurity career path presents a dynamic journey filled with diverse opportunities for professionals dedicated to safeguarding digital assets in our interconnected world. It typically commences with a foundational education in computer science, information technology, or cybersecurity, often complemented by specialized certifications like CompTIA Security+ or Certified Information Systems Security Professional (CISSP). Entry-level roles, such as cybersecurity analysts or junior administrators, immerse individuals in hands-on experiences, covering aspects like network security, vulnerability assessment, and incident response.
As professionals progress, they may choose to specialize in fields like penetration testing, ethical hacking, or security architecture. Certifications such as Certified Ethical Hacker (CEH) and CompTIA Security+ become pertinent for those seeking expertise. Leadership roles, such as cybersecurity managers or Chief Information Security Officers (CISOs), entail strategic planning, policy development, and the oversight of an organization’s comprehensive security posture.
Given the ever-evolving threat landscape, a commitment to continuous learning is vital. Cybersecurity professionals actively engage in ongoing training, participate in industry conferences, and contribute to the community. Fueled by persistent demand for skilled experts, the cybersecurity career path promises a challenging yet rewarding journey where individuals play a pivotal role in defending against cyber threats and fortifying the resilience of digital systems.
What are the roles and responsibilities of a Cyber Security expert?
The roles and responsibilities of a cyber security expert are as follows-
- Security Analyst
– Monitor security alerts and incidents.
– Conduct security assessments and risk analysis.
– Implement security measures and best practices.
- Security Engineer
– Design and implement security systems and architectures.
– Configure and manage security tools and technologies.
– Conduct penetration testing and vulnerability assessments.
- Incident Responder
– Investigate and respond to security incidents.
– Develop and implement incident response plans.
– Coordinate with relevant teams during security incidents.
- Penetration Tester (Ethical Hacker)
– Identify vulnerabilities in systems and applications.
– Conduct penetration tests to assess security posture.
– Provide recommendations to address security weaknesses.
- Security Consultant
– Advise clients on cybersecurity strategies.
– Conduct security assessments and audits.
– Develop and deliver cybersecurity training programs.
- Security Architect
– Design and build secure information systems.
– Define security requirements for projects.
– Ensure compliance with security policies and standards.
- Security Manager
– Oversee the organization’s overall security program.
– Develop and implement security policies and procedures.
– Manage security teams and resources.
- Chief Information Security Officer (CISO)
– Provide strategic leadership for cybersecurity.
– Align security initiatives with business objectives.
– Represent cybersecurity concerns to executive leadership.
- Security Operations Center (SOC) Analyst
– Monitor security alerts and events in real time.
– Investigate and respond to security incidents.
– Collaborate with other teams for incident resolution.
- Security Compliance Analyst
– Ensure compliance with industry regulations and standards.
– Conduct compliance assessments and audits.
– Develop and maintain compliance documentation.
- Security Trainer and Educator
– Provide cybersecurity training to employees.
– Develop educational materials on security best practices.
– Raise awareness about cybersecurity risks.
- Security Software Developer
– Develop secure software and applications.
– Implement security features in software development.
– Conduct code reviews for security vulnerabilities.
- Cyber Threat Intelligence Analyst
– Collect and analyze threat intelligence data.
– Provide insights on emerging threats.
– Support incident response with threat intelligence.
- Cryptographer
– Develop cryptographic algorithms and protocols.
– Ensure the security of data encryption.
– Research and analyze cryptographic techniques.
- Forensic Analyst
– Investigate cybercrime and security incidents.
– Collect and analyze digital evidence.
– Prepare reports for legal proceedings.
What cybersecurity skills are required in the digital era?
The cybersecurity skills required in the digital era are as follows-
- Technical Proficiency
- Network Security: Understanding network protocols, firewalls, and intrusion detection/prevention systems.
- Operating Systems: Proficiency in securing Windows, Linux, and macOS.
- Coding and Scripting: Knowledge of Python, Java, or PowerShell for automation and scripting.
- Cybersecurity Fundamentals
- Encryption Techniques: Familiarity with cryptographic algorithms, SSL/TLS, and secure key management.
- Security Protocols: Understanding protocols like IPsec, and DNSSEC for secure communication.
- Security Tools and Technologies
- Firewalls and IDS/IPS: Configuring and managing firewalls, intrusion detection, and prevention systems.
- Antivirus and Anti-Malware: Grasping tools for detecting, preventing, and removing malicious software.
- Incident Response and Forensics
- Incident Handling: Effectively responding to and managing security incidents.
- Digital Forensics: Skills in collecting, analyzing, and preserving digital evidence.
- Vulnerability Assessment and Penetration Testing
- Ethical Hacking: Knowledge of penetration testing tools to identify and address vulnerabilities.
- Vulnerability Management: Conducting regular assessments and prioritizing vulnerabilities.
- Identity and Access Management (IAM)
- Authentication and Authorization: Understanding multi-factor authentication, and role-based access control (RBAC).
- Security Architecture and Design
- Secure System Design: Knowledge of designing secure systems and architectures.
- Security Frameworks: Familiarity with cybersecurity frameworks such as NIST, ISO 27001, or CIS.
- Cloud Security
- Cloud Architecture: Understanding secure cloud environments and services.
- Identity Management in the Cloud: Skills in managing identities and access in cloud platforms.
- Security Awareness and Training
- Education Programs: Creating and delivering cybersecurity awareness programs for employees.
- Communication Skills: Effectively conveying complex security concepts to non-technical stakeholders.
- Data Protection and Privacy
- Data Encryption: Implementing encryption measures to protect sensitive data.
- Privacy Laws and Regulations: Knowledge of data protection laws and compliance requirements.
- Security Governance and Risk Management
- Risk Assessment: Assessing and managing risks to an organization’s information assets.
- Security Policies and Procedures: Developing and enforcing security policies.
- Soft Skills
- Problem-Solving: Critical thinking and problem-solving skills for complex security challenges.
- Adaptability: Ability to adapt to new technologies and evolving threat landscapes.
- Continuous Learning and Updating
- Stay Informed: Proactively stay updated on the latest cybersecurity trends, threats, and technologies.
- Collaboration and Teamwork
- Interpersonal Skills: Effectively collaborating with cross-functional teams and external partners.
- Legal and Ethical Understanding
- Compliance: Understanding and adhering to legal and ethical standards in cybersecurity.
- Ethical Hacking: Conducting security testing within ethical boundaries.
- Strategic Thinking
- Alignment with Business Objectives: Integrating cybersecurity strategies with overall business goals.
What are the common cyber security challenges in IT?
The common cyber security challenges in IT are as follows-
- Sophisticated Cyber Threats
The rise of advanced cyber threats, such as ransomware, zero-day exploits, and nation-state attacks, poses substantial challenges for IT security.
- Rapidly Evolving Technology
The swift evolution of technologies like cloud computing, IoT (Internet of Things), and AI introduces novel attack vectors and vulnerabilities, necessitating continuous adaptation and security measures.
- Insider Threats
Insider threats, whether inadvertent or malicious, present a significant challenge. Employees with access to sensitive information may inadvertently compromise security or act with malicious intent.
- Inadequate Security Awareness
A lack of awareness and understanding of cybersecurity best practices among employees can result in unintentional security breaches, such as falling victim to phishing attacks or using weak passwords.
- Supply Chain Vulnerabilities
Interconnected supply chains expose organizations to vulnerabilities in third-party vendors or service providers, leading to potential security breaches.
- Skill Shortages
A shortage of skilled cybersecurity professionals challenges organizations in building and maintaining robust security measures, potentially hindering timely responses to emerging threats.
- Legacy Systems and Outdated Software
The persistent use of legacy systems and outdated software poses risks, as these systems may have known vulnerabilities no longer patched or supported.
- Inadequate Security Policies
Poorly defined or outdated security policies and procedures may result in a lack of clarity regarding security expectations, increasing vulnerabilities.
- Incident Response Challenges
Organizations may struggle with establishing and implementing effective incident response plans, impeding their ability to respond promptly and effectively to security incidents.
- Compliance and Regulatory Issues
Achieving and maintaining compliance with various cybersecurity regulations and standards can be challenging, particularly for organizations operating across multiple jurisdictions.
- Data Privacy Concerns
The increasing focus on data privacy, coupled with regulations like GDPR, presents challenges in ensuring secure and compliant handling of personal and sensitive data.
- Limited Budgets
Many organizations face budget constraints that may limit their ability to invest in the latest cybersecurity technologies and training programs, leaving them vulnerable to sophisticated threats.
- Mobile Security Risks
The widespread use of mobile devices in the workplace introduces additional security risks, given these devices may be more susceptible to loss, theft, or unauthorized access.
- Cloud Security Challenges
Securing cloud environments poses unique challenges, including misconfigurations, shared responsibility models, and the need for specialized cloud security expertise.
- Global and Geopolitical Threats
Organizations may confront cybersecurity challenges influenced by geopolitical tensions, with attacks orchestrated by nation-states or hacktivist groups.
What are the goals and objectives of cyber security?
The goals and objectives of cyber security are as follows-
- Confidentiality
- It employs encryption to shield sensitive information from unauthorized access.
- It enforces stringent access controls, restricting information access based on user permissions.
- Integrity
- It implements data validation mechanisms to detect and prevent unauthorized alterations.
- It uses checksums and digital signatures to verify the integrity of data throughout its lifecycle.
- Availability
- It establishes redundancy measures to mitigate the impact of system failures.
- It develops robust disaster recovery plans for swift system recovery post-incidents.
- Authentication
- It implements strong authentication methods like passwords, biometrics, and multi-factor authentication.
- It regularly reviews and updates authentication protocols to ensure identity verification.
- Authorization
- It implements access controls and role-based access control (RBAC) to grant appropriate access privileges.
- It periodically reviews and updates user permissions based on job roles.
- Non-Repudiation
- It implements digital signatures to verify the origin and authenticity of transactions.
- It maintains comprehensive transaction logs and audit trails to prevent denial of actions.
- Risk Management
- It conducts regular risk assessments to identify potential vulnerabilities.
- It develops and implements risk mitigation strategies for information assets.
- Security Policies
- It develops and communicates acceptable use policies to define guidelines for cybersecurity.
- It establishes data protection policies and incident response plans.
- Incident Response
- It develops and tests incident response plans for a structured approach to addressing cybersecurity incidents.
- It establishes communication protocols and forensic analysis procedures for effective incident management.
- Security Awareness and Training
- It implements training programs regularly to educate users about cybersecurity best practices.
- It conducts awareness campaigns to keep users informed about evolving threats.
- Cryptography
- It implements encryption algorithms for safeguarding data in transit and at rest.
- It ensures secure key management practices to maintain cryptographic integrity.
- Firewalls and Network Security
- It deploys firewalls and intrusion detection/prevention systems for controlling network traffic.
- It designs and maintains a secure network architecture to mitigate potential threats.
- Security Architecture
- It implements layered security measures and a defence-in-depth strategy for robust security.
- It regularly assesses and updates security architecture to adapt to evolving threats.
- Security Governance
- It gains leadership commitment to cybersecurity initiatives for effective governance.
- It develops and enforces cybersecurity policies, overseeing comprehensive security programs.
- Vulnerability Management
- It conducts regular vulnerability assessments to identify and prioritize vulnerabilities.
- It implements patch management and security updates for effective risk mitigation.
What are the future cyber security trends?
The future cyber security trends are as follows-
- Zero Trust Architecture
The Zero Trust model, assuming no trust by default and verifying every user and device accessing the network, is expected to gain prominence. This approach enhances security in an increasingly perimeterless and cloud-centric environment.
- AI and Machine Learning in Cybersecurity
The integration of artificial intelligence (AI) and machine learning (ML) is likely to continue, aiding in threat detection, behavioural analysis, and automated response. This trend enhances the ability to identify and respond to sophisticated cyber threats.
- Extended Detection and Response (XDR)
XDR platforms, integrating various security solutions to provide more comprehensive threat detection and response capabilities, are expected to become more prevalent. XDR goes beyond traditional endpoint detection and response (EDR) solutions.
- Cloud Security Posture Management (CSPM)
With the increasing adoption of cloud services, there is a growing focus on Cloud Security Posture Management tools. These tools help organizations ensure the secure configuration of their cloud environments and prevent misconfigurations that could lead to vulnerabilities.
- 5G Security Challenges
The rollout of 5G networks introduces new security challenges, including increased attack surfaces and potential vulnerabilities. Ensuring the security of 5G infrastructure will be a priority.
- Ransomware Defense Strategies
As ransomware attacks continue to evolve, organizations are expected to invest more in robust backup strategies, incident response planning, and security awareness training to defend against and recover from ransomware incidents.
- Supply Chain Security
The SolarWinds incident highlighted the importance of securing the software supply chain. Organizations are likely to focus on assessing and improving the security of third-party vendors and software providers.
- IoT Security
With the proliferation of Internet of Things (IoT) devices, ensuring the security of these interconnected devices will be crucial. This includes implementing security measures in both consumer and industrial IoT applications.
- Quantum-Safe Cryptography
The advent of quantum computing poses a potential threat to current cryptographic methods. The adoption of quantum-safe or quantum-resistant cryptographic algorithms is anticipated to address this concern.
- Biometric Security Advances
Biometric authentication methods, such as facial recognition and fingerprint scanning, are expected to advance, providing more secure and user-friendly authentication options.
- Cybersecurity Regulations and Compliance
Governments and regulatory bodies are likely to introduce and enforce more cybersecurity regulations to enhance data protection and privacy. Organizations will need to stay compliant with evolving legal requirements.
- Human-Centric Security
Recognizing the role of human factors in cybersecurity, there will be a focus on human-centric security measures, including user education, awareness training, and behavioural analysis.
- Edge Computing Security
With the rise of edge computing, securing decentralized infrastructure at the edge of networks will become a priority to protect sensitive data closer to its source.
- Cybersecurity Skills Development
The demand for skilled cybersecurity professionals is expected to continue growing. Organizations and individuals will emphasize ongoing skills development and training to address the workforce shortage.
What is Data Science?
Data Science is a multidisciplinary field that integrates methods, processes, and systems to derive meaningful insights from structured and unstructured data. It combines statistical analysis, machine learning, data engineering, and domain expertise to identify patterns, trends, and correlations within extensive datasets. The primary objective of data science is to produce actionable and data-driven insights that guide decision-making and address intricate problems.
The data science workflow typically includes the collection, cleaning, and preprocessing of data, followed by exploratory data analysis to comprehend underlying patterns. Machine learning models are then employed to create predictive or prescriptive models, depending on specific goals. Data scientists utilize programming languages such as Python or R, along with various tools and frameworks, to efficiently manipulate and analyze data.
Data Science is applied across diverse industries, including finance, healthcare, marketing, and technology. It plays a pivotal role in streamlining business processes, enhancing product development, and refining decision-making by transforming raw data into valuable knowledge. As technology progresses, data science undergoes continuous evolution, making substantial contributions to innovation and complex problem-solving in the contemporary data-driven era.
What is the importance of Data Science?
The significance of Data Science lies in its transformative ability to extract valuable insights from intricate datasets. The importance of cyber security can be understood as follows-
- Informed Decision-Making
Data Science empowers organizations to make strategic decisions by offering actionable insights, leading to improved business outcomes.
- Business Optimization
Through data analysis, businesses identify areas for improvement, optimizing processes for increased efficiency and cost savings.
- Predictive Analytics
Data Science enables predictive modelling, allowing organizations to foresee trends, customer behaviours, and market changes, facilitating proactive decision-making.
- Innovation and Product Development
Data-driven insights guide innovation in product development, aligning with customer preferences and market trends to maintain competitiveness.
- Personalized Customer Experiences
Data analysis helps understand individual customer preferences, enabling personalized products, services, and marketing for enhanced satisfaction.
- Fraud Detection and Security
Data Science is crucial for detecting anomalies and patterns associated with fraudulent activities, and ensuring the security of financial transactions and sensitive information.
- Healthcare Advancements
In healthcare, Data Science contributes to personalized medicine, predictive diagnostics, and treatment optimization, improving patient outcomes.
- Scientific Research and Exploration
Data Science plays a pivotal role in scientific research, aiding the analysis of large datasets and making discoveries in fields like astronomy, genetics, and environmental science.
- Economic and Social Impact
Data Science has far-reaching effects on economic growth and societal progress, guiding governments, businesses, and non-profits to address challenges and drive positive change.
- Efficient Resource Allocation
Organizations optimize resource allocation with data-driven insights, impacting human resources, budget allocation, and overall planning.
- Continuous Learning and Adaptation
Data Science fosters a culture of continuous learning, providing real-time feedback and insights to keep organizations agile and responsive.
- Data Monetization
Data Science identifies opportunities for data monetization, unlocking additional revenue streams through selling insights or collaborative ventures.
What is the scope of Data Science?
- Rising Demand
The scope of Data Science is broadening swiftly owing to the escalating need for professionals proficient in analyzing and interpreting extensive datasets to guide business decisions.
- Relevance Across Industries
Data Science manifests its applicability across a spectrum of industries, encompassing finance, healthcare, marketing, e-commerce, and technology. This underscores its extensive and adaptable scope.
- Predictive Analytics Utility
Organizations employ Data Science for predictive analytics, enabling them to anticipate trends, comprehend customer behaviours, and adapt to market changes, thereby facilitating proactive decision-making.
- Driving Innovation
Acting as a catalyst for innovation, Data Science directs product development, refines processes, and heightens overall operational efficiency. It assumes a pivotal role as a primary driver of innovation in diverse sectors.
- Personalization Facilitation
The capability to scrutinize individual customer preferences empowers the creation of personalized products, services, and marketing strategies. This underscores Data Science’s pivotal role in augmenting customer experiences.
- Advancements in Healthcare
Within the healthcare sector, Data Science contributes significantly to the realms of personalized medicine, predictive diagnostics, and treatment optimization, culminating in enhanced patient outcomes.
- Crucial Role in Fraud Detection
Data Science assumes a critical role in identifying anomalies and patterns associated with fraudulent activities, ensuring the security and integrity of financial transactions.
- Constant Evolution
The field undergoes perpetual evolution propelled by technological advancements and the emergence of novel data sources, indicating an ever-expanding scope for Data Science professionals.
- Abundant Career Prospects
The escalating dependence on data-driven insights creates a heightened demand for adept data scientists, presenting abundant career opportunities and promising job prospects.
- Integral to Decision-Making
Data Science has become inseparable from organizational decision-making processes, underscoring its widespread scope in influencing both strategic and operational choices.
What are the core concepts of Data Science?
The core concepts of Data Science are as follows-
- Data Collection
Gathering pertinent data from diverse sources, ensuring it is thorough, precise, and reflective of the problem under consideration.
- Data Cleaning and Preprocessing
Managing missing or erroneous data, standardizing formats, and transforming data into a usable state for analysis.
- Exploratory Data Analysis (EDA)
Analyzing and visualizing data to discern patterns, distributions, and relationships, offering insights into the dataset’s structure.
- Statistical Analysis
Applying statistical methods to recognize trends, make predictions, and draw meaningful conclusions from data.
- Machine Learning
Utilizing algorithms and models for systems to learn from data, make predictions, and execute tasks without explicit programming.
- Feature Engineering
Selecting, transforming, or creating new features from raw data to enhance the performance of machine learning models.
- Model Evaluation and Validation
Assessing machine learning model performance, ensuring effective generalization to new, unseen data.
- Big Data Technologies
Familiarity with tools and frameworks designed for efficient handling of large and complex datasets, such as Apache Hadoop and Apache Spark.
- Data Visualization
Representing data visually through charts, graphs, and dashboards to effectively communicate findings.
- Domain Knowledge
Understanding the specific industry or domain to contextualize data analysis and generate meaningful insights.
- Ethics and Privacy
Acknowledging ethical considerations and privacy concerns in handling and analyzing sensitive data.
- Communication Skills
Articulating findings and insights to non-technical stakeholders, facilitating informed decision-making.
- Coding and Programming
Proficiency in programming languages like Python or R for data manipulation, analysis, machine learning model implementation, and task automation.
- Data Governance
Establishing policies and procedures for managing data throughout its lifecycle, ensuring quality, security, and compliance.
- Reproducibility
Implementing practices allowing others to reproduce analyses and results, promoting transparency and reliability.
- Experimentation and Hypothesis Testing
Conducting controlled experiments and hypothesis testing to validate assumptions and draw conclusions based on statistical significance.
- Time Series Analysis
Understanding and analyzing data collected over time to identify patterns, and trends, and forecast future values.
What are the key technologies in Data Science?
The key technologies in Data Science are as follows-
- Programming Languages
Python: Widely used for data manipulation, analysis, and machine learning.
R: Commonly employed for statistical analysis and data visualization.
- Data Manipulation and Analysis
Pandas: A Python library for data manipulation and analysis.
NumPy: Used for numerical operations and mathematical functions.
- Machine Learning Frameworks
Scikit-learn: A versatile machine learning library in Python.
TensorFlow and PyTorch: Deep learning frameworks for building and training neural networks.
- Data Visualization
Matplotlib and Seaborn: Python libraries for creating static, interactive, and statistical visualizations.
Tableau: A powerful tool for creating interactive and shareable dashboards.
- Big Data Technologies
Apache Hadoop: A framework for distributed storage and processing of large datasets.
Apache Spark: Enables fast and distributed data processing.
- Database Technologies
SQL (Structured Query Language): Essential for managing and querying relational databases.
NoSQL Databases (MongoDB, Cassandra): Suited for handling unstructured or semi-structured data.
- Data Mining and Exploration
RapidMiner: An open-source platform for data science and machine learning.
Weka: A collection of machine learning algorithms for data mining tasks.
- Cloud Computing Services
AWS, Azure, and Google Cloud: Cloud platforms offering services for scalable and on-demand computing, storage, and analytics.
- Statistical Analysis
Jupyter Notebooks: Interactive notebooks for combining code, visualizations, and narrative text.
RStudio: An integrated development environment for R.
- Natural Language Processing (NLP)
NLTK (Natural Language Toolkit): A Python library for NLP tasks.
Spacy: An open-source NLP library for advanced natural language processing.
- Version Control
Git: A distributed version control system for tracking changes in code and collaborative development.
- Containerization
Docker: Enables packaging and deploying applications and dependencies in containers.
- Collaboration Tools
GitHub and GitLab: Platforms for version control and collaborative software development.
- Workflow Automation
Apache Airflow: A platform to programmatically author, schedule, and monitor workflows.
- GPU Acceleration
CUDA (Compute Unified Device Architecture): A parallel computing platform for utilizing GPUs in data processing and machine learning.
How to become a Data Science professional? - Data Scientist
Embarking on a career in Data Science involves a blend of education, practical exposure, and ongoing learning. Here is a step-by-step guide to guide you on your journey:
- Educational Background
Cultivate a robust base in mathematics, statistics, and computer science. A bachelor’s degree in related fields like computer science, mathematics, or physics serves as a solid starting point.
- Learn Programming Languages
Attain proficiency in pivotal programming languages for Data Science, such as Python and R. Familiarize yourself with essential libraries like NumPy, Pandas, and Scikit-learn.
- Acquire Data Analysis Skills
Master data manipulation, cleansing, and exploratory data analysis (EDA) techniques. Practice handling datasets and extracting meaningful insights.
- Study Machine Learning
Grasp fundamental machine learning concepts, algorithms, and models. Delve into both supervised and unsupervised learning techniques, leveraging online courses, books, and tutorials.
- Deepen Statistical Knowledge
Elevate your comprehension of statistical methods essential for Data Science, including hypothesis testing, regression analysis, and probability.
- Explore Data Visualization
Hone expertise in data visualization tools and techniques. Learn to articulate insights effectively through tools like Matplotlib, Seaborn, and Tableau.
- Build a Strong Foundation in Big Data Technologies
Familiarize yourself with big data tools and frameworks, including Apache Hadoop and Apache Spark. Understand the nuances of processing and analyzing extensive datasets.
- Develop Database Skills
Master working with databases and SQL. Gain proficiency in both relational databases and NoSQL databases like MongoDB.
- Gain Practical Experience
Apply your theoretical knowledge by working on real-world projects. Engage in Kaggle competitions, contribute to open-source projects, or pursue personal projects to showcase your skills.
- Network and Connect
Attend Data Science meetups, conferences, and networking events. Forge connections with professionals in the field, participate in online communities, and seek mentorship to glean insights and advice.
- Build a Strong Portfolio
Showcase your projects, skills, and accomplishments in a portfolio. Provide detailed explanations of your projects, outlining the problems you addressed and the impact of your work.
- Apply for Internships and Entry-Level Positions
Garner practical experience through internships or entry-level roles. Hands-on exposure is invaluable for refining your resume and enhancing your practical skills.
- Stay Updated and Engage in Continuous Learning
Recognize the dynamic nature of the Data Science field. Stay abreast of emerging technologies, tools, and industry trends, and foster continuous learning through online courses, webinars, and workshops.
- Develop Soft Skills
Enhance your communication and collaboration skills. Effective communication is pivotal as data scientists often need to convey complex findings to non-technical stakeholders.
Discuss the Data Science career path.
Embarking on a Data Science career is a dynamic and varied journey, presenting professionals with numerous opportunities to shape data-driven decision-making across diverse industries. Typically initiated by a solid educational foundation in mathematics, statistics, and computer science, often through a bachelor’s degree, aspirants then immerse themselves in mastering essential programming languages like Python and R. This includes honing skills in data manipulation, statistical analysis, and machine learning techniques.
As individuals establish their foundational skills, they progress to gaining practical experience through real-world projects, internships, or entry-level positions. Further educational pursuits, such as obtaining a master’s degree or relevant certifications in Data Science, contribute to increased expertise and competitiveness in the job market. Networking within the Data Science community, attending conferences, and continuous learning are pivotal for staying abreast of evolving industry trends.
The career path further diverges into specialized roles such as Data Analyst, Machine Learning Engineer, and Data Engineer, each demanding specific skill sets. With accumulated experience, professionals may advance into leadership positions like Data Science Manager or Chief Data Officer, guiding strategic decisions grounded in data insights. The Data Science career trajectory underscores a commitment to lifelong learning, adaptability, and a fervour for utilizing data to solve intricate problems and propel innovation.
What are the roles and responsibilities of a Data Science expert?
The roles and responsibilities of a Data Science expert are as follows-
- Problem-Solving
Articulate and understand the business problems or objectives that data analysis aims to solve.
- Data Collection
Gather pertinent data from diverse sources, ensuring its quality, completeness, and accuracy.
- Data Cleaning and Preprocessing
Clean and preprocess data to handle missing values, and outliers, and ensure it is in a suitable format for analysis.
- Exploratory Data Analysis (EDA)
Conduct exploratory data analysis to uncover underlying patterns, relationships, and distributions within the data.
- Statistical Analysis
Apply statistical methods to identify trends, patterns, and correlations in the data.
- Machine Learning Modeling
Develop and implement machine learning models based on specific problem requirements, selecting appropriate algorithms and techniques.
- Feature Engineering
Choose, transform, or create features to enhance the performance of machine learning models.
- Model Evaluation and Validation
Assess the performance of machine learning models, ensuring their generalization to new, unseen data.
- Data Visualization
Create visualizations to effectively communicate findings and insights to both technical and non-technical stakeholders.
- Communication Skills
Effectively convey complex technical concepts and findings to non-technical stakeholders, contributing to informed decision-making.
- Continuous Learning
Stay abreast of emerging technologies, tools, and methodologies within the evolving field of Data Science.
- Collaboration
Collaborate with cross-functional teams, including data engineers, business analysts, and domain experts, integrating data-driven insights into business strategies.
- Experimentation and Hypothesis Testing
Conduct controlled experiments and hypothesis testing to validate assumptions, drawing conclusions based on statistical significance.
- Ethics and Privacy
Adhere to ethical considerations and privacy regulations while handling and analyzing sensitive data.
- Model Deployment
Deploy machine learning models into production environments, ensuring scalability and efficiency.
- Monitoring and Maintenance
Continuously monitor and maintain deployed models, updating them as necessary to adapt to changing data patterns.
- Training and Mentorship
Provide training and mentorship to junior members of the data science team, fostering a collaborative and knowledge-sharing environment.
- Strategic Decision Support
Assist in guiding strategic decisions by providing insights and recommendations based on thorough data analysis.
What Data Science skills are required in the digital era?
The Data Science skills required in the digital era are as follows-
- Programming Languages
Mastery of languages like Python and R for tasks such as data manipulation, analysis, and machine learning.
- Statistical Analysis
Robust statistical skills to interpret data, recognize patterns, and draw insightful conclusions.
- Machine Learning
Comprehensive understanding of machine learning algorithms and techniques for predictive modelling and pattern recognition.
- Data Cleaning and Preprocessing
Expertise in cleansing and preprocessing data, addressing missing values, and outliers, and ensuring data quality.
- Big Data Technologies
Familiarity with big data tools like Apache Hadoop and Apache Spark for efficient processing and analysis of large datasets.
- Data Visualization
Proficiency in crafting compelling visualizations using tools like Matplotlib, Seaborn, or Tableau to effectively communicate insights.
- Cloud Computing
Knowledge of cloud platforms (AWS, Azure, Google Cloud) for scalable and on-demand computing, storage, and analytics.
- Database Management
Skills in working with databases and SQL, coupled with an understanding of NoSQL databases for diverse data types.
- Natural Language Processing (NLP)
Understanding and application of NLP techniques for processing and analyzing human language data.
- Feature Engineering
Ability to select, transform, or create features to enhance machine learning model performance.
- Version Control
Proficiency in version control systems like Git for tracking changes in code and facilitating collaboration with teams.
- Ethical Considerations
Awareness of ethical considerations and privacy issues related to handling sensitive data.
- Continuous Learning
A commitment to ongoing learning to stay abreast of emerging technologies, tools, and industry trends.
- Collaboration and Communication
Strong collaboration and communication skills for effective teamwork with cross-functional units and clear communication of findings to non-technical stakeholders.
- Experimentation and Hypothesis Testing
Capability to design and conduct controlled experiments, validate assumptions, and draw conclusions based on statistical significance.
- Cybersecurity Awareness
Understanding of fundamental cybersecurity principles to ensure data security and integrity.
- Business Acumen
Insight into business processes and objectives, aligning data science initiatives with organizational goals.
- Problem-Solving Skills
Strong analytical and problem-solving skills to address complex business challenges through data-driven approaches.
- Automation and Workflow
Knowledge of tools like Apache Airflow for workflow automation, streamlining data processes, and analyses.
- Critical Thinking
Ability to think critically and approach data problems with a strategic mindset.
What are the common Data Science challenges?
The common Data Science challenges are as follows-
- Data Quality
Ensuring the precision, correctness, and completeness of data proves to be a formidable challenge. Inconsistent or incomplete datasets can compromise the accuracy of insights and the reliability of models.
- Data Integration
The amalgamation of data from disparate sources, each with unique formats and structures, poses a challenge. Integration issues may emerge, affecting the ability to derive cohesive insights.
- Data Privacy and Security
Safeguarding sensitive data and ensuring compliance with privacy regulations presents an ongoing challenge. Striking a balance between data utility and privacy concerns remains pivotal.
- Lack of Domain Knowledge
A profound comprehension of the specific domain or industry context is indispensable for effective data analysis. A deficiency in domain knowledge can impede the interpretation and application of analytical results.
- Scalability
The handling of large datasets introduces scalability challenges. Ensuring that algorithms and processes can adeptly manage increasing data volumes is a perpetual concern.
- Model Interpretability
Grappling with the interpretation of intricate machine learning models, especially when elucidating results to non-technical stakeholders, is a nuanced challenge. Balancing model accuracy with interpretability is of paramount importance.
- Keeping Pace with Technology
The rapid evolution of Data Science tools and technologies demands perpetual skill updates. Staying abreast of the latest advancements can be an exacting task.
- Resource Constraints
Limited computational resources and processing power may constrain the execution of intricate analyses or the training of advanced machine-learning models.
- Overfitting and Underfitting
Striking a delicate balance between overfitting (capturing noise in the data) and underfitting (oversimplifying the model) is a recurrent challenge in machine learning model development.
- Communication Gap
Effectively conveying findings to non-technical stakeholders poses a challenge. Bridging the communication gap between data scientists and business decision-makers is pivotal for actionable insights.
- Bias in Data and Models
Addressing bias in both the data and the models is indispensable to ensure fairness and prevent the perpetuation of existing biases in decision-making processes.
- Deployment Challenges
Transitioning from a successful model in a development environment to deployment in a production environment introduces challenges related to integration, scalability, and real-time processing.
- Measuring Business Impact
Gauging the tangible impact of Data Science initiatives on business outcomes and ROI is a complex challenge. Aligning analytics with key performance indicators (KPIs) assumes critical significance.
- Resistance to Change
Facing resistance within organizations to adopt data-driven decision-making can impede the seamless integration of Data Science insights into business processes.
- Data Governance
Establishing and perpetuating effective data governance practices, including data quality standards and compliance, remains an ongoing challenge.
What are the goals and objectives of Data Science?
The goals and objectives of Data Science are as follows-
- Informed Decision-Making
Equip organizations with actionable insights from data analysis, facilitating informed and strategic decision-making.
- Business Optimization
Identify opportunities for enhancing processes, reducing costs, and improving overall operational efficiency by analyzing and streamlining business workflows.
- Predictive Analytics
Develop models that predict future trends, customer behaviours, and market changes, empowering proactive decision-making and planning.
- Innovation and Product Development
Foster innovation by leveraging data-driven insights to guide the development of new products, enhance existing ones, and maintain competitiveness in the market.
- Personalized Customer Experiences
Utilize data analysis to comprehend individual customer preferences and behaviours, enabling the customization of products, services, and marketing strategies for heightened customer satisfaction.
- Fraud Detection and Security
Apply Data Science techniques to detect anomalies and patterns linked to fraudulent activities, ensuring the security and integrity of financial transactions and sensitive information.
- Healthcare Advancements
Contribute to healthcare progress through personalized medicine, predictive diagnostics, and treatment optimization, ultimately leading to improved patient outcomes.
- Scientific Research and Exploration
Assist researchers in analyzing extensive datasets, conducting simulations, and making breakthroughs in fields such as astronomy, genetics, and environmental science.
- Economic and Social Impact
Leverage data to address societal challenges, inform policies, and contribute to economic growth, fostering positive societal impact.
- Efficient Resource Allocation
Optimize resource allocation by using data-driven insights to pinpoint areas of high impact, whether in human resources, budget allocation, or overall organizational planning.
- Continuous Learning and Adaptation
Instil a culture of continuous learning and adaptation by providing real-time feedback and insights, enabling organizations to stay agile and responsive to changing environments.
- Data Monetization
Identify opportunities for organizations to monetize their data, whether through selling insights, collaborating with partners, or creating data-driven products.
What are the future Data Science trends?
The future Data Science trends are as follows-
- AI Integration
Deepening the fusion of artificial intelligence (AI) and machine learning within Data Science workflows, amplifying predictive analytics and decision-making capabilities.
- Explainable AI
Prioritizing the development of models with heightened interpretability and transparency to address concerns regarding the opaque nature of intricate algorithms.
- Automated Machine Learning (AutoML)
Witnessing increased adoption of AutoML tools, empowering non-experts to construct machine learning models and streamline the model development process.
- Augmented Analytics
Integrating AI and machine learning into analytics tools, furnishing users with automated insights and recommendations, diminishing the necessity for manual analysis.
- Edge Computing for Data Processing
Embracing the processing and analysis of data closer to the source (edge computing), mitigating latency and tackling challenges linked to real-time analytics.
- Responsible AI and Ethical Data Science
Intensifying attention on ethical considerations, fairness, and accountability in AI and Data Science practices, prompted by societal concerns and regulatory frameworks.
- Advanced Natural Language Processing (NLP)
Advancing NLP techniques to enable more nuanced language understanding, sentiment analysis, and interaction with unstructured text data.
- Blockchain for Data Security
Experiencing heightened adoption of blockchain technology to fortify data security, integrity, and transparency, especially in applications such as data sharing and collaborative research.
- DataOps Practices
Integrating DataOps practices to enhance collaboration, communication, and automation throughout the end-to-end data lifecycle, thereby boosting efficiency and reducing time-to-insights.
- Exponential Growth of IoT Data
Observing a surge in data volume due to the proliferation of Internet of Things (IoT) devices, necessitating advanced analytics solutions for meaningful insights.
- Exascale Computing for Big Data
Harnessing the capabilities of exascale computing to efficiently process and analyze massive datasets, addressing challenges related to scalability and computational power.
- Exponential Increase in Unstructured Data Analysis
Recognizing the escalating importance of analyzing unstructured data like images, videos, and audio, facilitated by advancements in computer vision and audio processing.
- Exponential Increase in Unstructured Data Analysis
Acknowledging the rising significance of analyzing unstructured data, including images, videos, and audio, facilitated by advancements in computer vision and audio processing.
- Human Augmentation with AI
Exploring AI-driven technologies to enhance human decision-making, problem-solving, and data analysis capabilities.
- Quantum Computing Applications
Investigating potential applications of quantum computing to address complex optimization problems and expedite certain types of machine learning algorithms.
Cyber Security vs Data Science - Explained
Cybersecurity vs Data Science represent distinct domains, each with its unique focus and objectives. Let us now understand the differences and similarities between the two in detail.
What is the difference between Cyber Security and Data Science?
The differences between Cyber Security and Data Science on different basis are as follows-
DIFFERENCE | CYBER SECURITY | DATA SCIENCED |
FOCUS AREA | Primarily centered on safeguarding digital systems, networks, and sensitive information from cyber threats and attacks. | Concentrates on extracting insights and knowledge from data using diverse techniques and methodologies. |
OBJECTIVE | Aims to protect the confidentiality, integrity, and availability of information and systems. | Aims to analyze and interpret data to derive actionable insights, inform decision-making, and solve complex problems. |
PRIMARY CONCERN | Mitigating risks related to unauthorized access, data breaches, malware, and other cyber threats. | Uncovering patterns, trends, and relationships within data to drive business decisions and innovations. |
SKILL SET | Requires skills in network security, cryptography, incident response, and vulnerability management. | Requires skills in statistics, programming, machine learning, and data visualization. |
TOOLS & TECHNOLOGIES | Involves tools for intrusion detection, firewalls, antivirus, and security information and event management (SIEM). | Involves tools for data manipulation (e.g., Python, R), machine learning frameworks, and data visualization tools. |
PREVENTIVE vs DESCRIPTIVE | Primarily preventive, focusing on measures to prevent and mitigate cyber threats. | Often descriptive, aiming to describe and understand patterns in historical data. |
ROLE IN DECISION-MAKING | Enables secure decision-making by protecting critical information assets. | Informs decision-making by providing insights derived from data analysis. |
LIFE CYCLE | Involves continuous monitoring, detection, and response to evolving cyber threats. | Encompasses data collection, cleaning, analysis, modelling, and interpretation. |
ETHICAL CONSIDERATIONS | Focuses on ensuring privacy, confidentiality, and ethical handling of sensitive information. | Addresses ethical considerations in data collection, usage, and potential biases in algorithms. |
REGULATORY COMPLIANCE | Adheres to regulations related to data protection and privacy. | Adheres to data governance and privacy regulations. |
OUTCOME MEASUREMENT | Success is often measured by the absence of security incidents and breaches. | Success is measured by the ability to provide actionable insights and improve decision-making. |
PROACTIVE vs REACTIVE | Often reactive, responding to and mitigating threats as they occur. | Proactive, seeking patterns and trends to anticipate future events. |
DATA HANDLING | Focuses on protecting data from unauthorized access and ensuring its integrity. | Involves handling and analyzing data to derive meaningful insights. |
JOB ROLES | Roles include cybersecurity analyst, penetration tester, and security architect. | Roles include data scientist, machine learning engineer, and data analyst. |
NATURE OF THREATS | Deals with external threats like hackers, malware, and cybercriminals. | Focuses on extracting value from data and addressing challenges within the data itself. |
REAL-TIME vs HISTORICAL | Often operates in real-time to detect and respond to immediate threats. | Analyzes historical data to identify patterns and trends. |
COST CONSIDERATIONS | Investment in prevention and detection mechanisms to avoid financial losses from breaches. | Investment in technology and talent for data analysis, contributing to strategic decision-making. |
INTERDISCIPLINARY NATURE | Involves aspects of computer science, information technology, and risk management. | Integrates computer science, statistics, and domain-specific knowledge. |
SCOPE OF IMPACT | Impacts the overall security and integrity of digital systems and networks. | Impacts decision-making, innovation, and operational efficiency within an organization. |
COLLABORATION WITH OTHER FIELDS | Collaborates with risk management, compliance, and legal teams. | Collaborates with business, marketing, and research teams for data-driven insights. |
What are the similarities between Cybersecurity and Data Science?
The similarities between Cybersecurity and Data Science are as follows-
- Data Handling
Both fields manage substantial volumes of data. Cybersecurity analyzes data to detect malicious patterns, while Data Science extracts insights for informed decision-making.
- Statistical Analysis
Statistical techniques play a role in both domains. Cybersecurity applies statistics for anomaly detection, while Data Science utilizes statistical methods for data analysis.
- Programming Skills
Proficiency in languages like Python and R is essential in both Cybersecurity and Data Science for tasks like scripting, automation, and analysis.
- Machine Learning
Machine learning is a shared tool. Cybersecurity deploys ML for threat detection, and Data Science employs it for predictive modelling and pattern recognition.
- Pattern Recognition
Both fields involve pattern recognition, albeit in different contexts. Cybersecurity identifies patterns signalling cyber threats, while Data Science detects patterns within data for insights.
- Data Visualization
Techniques for data visualization are integral to both Cybersecurity and Data Science, aiding in presenting complex information understandably.
- Predictive Analytics
Predictive analytics is a common thread. Cybersecurity predicts potential threats, and Data Science forecasts trends based on historical data.
- Ethical Considerations
Ethical considerations and responsible data handling are paramount in both fields, addressing sensitive data concerns in Cybersecurity and potential biases in Data Science models.
- Continuous Learning
Ongoing learning is essential for professionals in both Cybersecurity and Data Science, given the dynamic nature of their respective fields.
- Collaboration
Collaboration across teams is crucial. Cybersecurity collaborates with IT and management, and Data Science collaborates with business and research teams.
- Real-Time Monitoring
Real-time monitoring is a shared aspect. Cybersecurity monitors network activities instantly, and Data Science applications may require real-time analytics.
- Anomaly Detection
Anomaly detection is a common concept. Cybersecurity uses it for identifying unusual network behaviour, and Data Science applies it to find irregularities in datasets.
- Security Measures
Implementation of security measures is practised by professionals in both Cybersecurity and Data Science. Cybersecurity secures digital assets, and Data Science ensures data integrity and confidentiality.
- Interdisciplinary Nature
Both fields draw from an interdisciplinary pool, incorporating elements of computer science, mathematics, and domain-specific knowledge to address complex challenges.
- Decision Support
Contribution to decision-making is a shared goal. Cybersecurity provides insights for secure decisions, and Data Science offers data-driven insights for strategic and operational decisions.
In Data Science vs Cyber Security, which is better - Cybersecurity or Data Science?
Choosing between Cybersecurity and Data Science hinges on personal interests, career aspirations, and the specific contributions one aims to make in the technology sector. Cybersecurity appeals to those driven by the desire to safeguard digital assets, counter cyber threats, and uphold system integrity. Roles like cybersecurity analyst or penetration tester are central to preventing and responding to security incidents.
In contrast, Data Science attracts individuals fascinated by extracting insights from data to drive decision-making and tackle intricate problems. Roles like data scientist or machine learning engineer involve data analysis, predictive modelling, and algorithm development. Both fields play pivotal roles in the contemporary tech landscape, with Cybersecurity prioritizing security and protection and Data Science emphasizing data-driven decision-making and innovation. The optimal choice depends on individual preferences, skill sets, and the envisioned impact within the dynamic domains of cybersecurity or data science.
Explain - Data Science vs Cyber Security Which pays more?
Both Cyber Security and Data Science pay well if you have the right skills. The pay for both varies according to the region and job role. Let us understand the salary aspects for both.
Where can I learn Cybersecurity and Data Science in detail?
To learn the top cybersecurity skills in IT, you can choose Network Kings. Being one of the best ed-tech platforms, you will get to enjoy the following perks-
- Learn directly from expert engineers
- 24*7 lab access
- Pre-recorded sessions
- Live doubt-clearance sessions
- Completion certificate
- Flexible learning hours
- And much more.
What are the available job roles for Data Science and Cyber Security experts?
The top available job roles for Data Science experts are as follows-
- Data Scientist
- Machine Learning Engineer
- Data Analyst
- Business Intelligence Analyst
- Data Engineer
- Statistician
- Quantitative Analyst
- Operations Analyst
- Research Scientist
- Big Data Engineer
- Business Analyst
- Predictive Modeler
- AI Specialist
- Computer Vision Engineer
- Natural Language Processing (NLP) Engineer
- Deep Learning Engineer
- Data Architect
- Database Administrator
- Statistician
- Analytics Manager
The top available job roles for Cyber Security experts are as follows-
- Cybersecurity Analyst
- Information Security Officer
- Network Security Engineer
- Penetration Tester
- Security Consultant
- Incident Responder
- Security Architect
- Cryptographer
- Security Software Developer
- Vulnerability Assessor
- Chief Information Security Officer (CISO)
- Security Operations Center (SOC) Analyst
- Identity and Access Management (IAM) Specialist
- Threat Intelligence Analyst
- Security Auditor
- Security Compliance Analyst
- Security Awareness Training Specialist
- Malware Analyst
- Security Researcher
- Cloud Security Engineer
What are the salary aspects for Cybersecurity and Data Science professionals?
The salary aspects for a Cybersecurity professional in different countries are as follows-
- United States: USD 90,000 – USD 150,000 per year
- United Kingdom: GBP 40,000 – GBP 80,000 per year
- Canada: CAD 80,000 – CAD 120,000 per year
- Australia: AUD 80,000 – AUD 120,000 per year
- Germany: EUR 60,000 – EUR 100,000 per year
- Singapore: SGD 70,000 – SGD 110,000 per year
- France: EUR 50,000 – EUR 90,000 per year
- India: INR 8,00,000 – INR 20,00,000 per year
- Japan: JPY 6,000,000 – JPY 10,000,000 per year
- Brazil: BRL 100,000 – BRL 200,000 per year
- South Africa: ZAR 400,000 – ZAR 800,000 per year
- UAE: AED 150,000 – AED 300,000 per year
- Sweden: SEK 500,000 – SEK 900,000 per year
- Netherlands: EUR 60,000 – EUR 100,000 per year
- Switzerland: CHF 100,000 – CHF 150,000 per year
- China: CNY 200,000 – CNY 500,000 per year
- South Korea: KRW 60,000,000 – KRW 120,000,000 per year
- Mexico: MXN 500,000 – MXN 1,000,000per year
- Russia: RUB 2,000,000 – RUB 5,000,000 per year
- Malaysia: MYR 80,000 – MYR 150,000 per year
The salary aspects for a Data Science professional in different countries are as follows-
- United States: USD 90,000 – USD 150,000 per year
- United Kingdom: GBP 40,000 – GBP 80,000 per year
- Canada: CAD 70,000 – CAD 120,000 per year
- Germany: EUR 50,000 – EUR 90,000 per year
- Australia: AUD 80,000 – AUD 130,000 per year
- Netherlands: EUR 50,000 – EUR 90,000 per year
- Singapore: SGD 70,000 – SGD 120,000 per year
- France: EUR 45,000 – EUR 85,000 per year
- Switzerland: CHF 100,000 – CHF 150,000 per year
- Sweden: SEK 500,000 – SEK 800,000 per year
- India: INR 6,00,000 – INR 20,00,000 per year
- Brazil: BRL 80,000 – BRL 150,000 per year
- South Africa: ZAR 400,000 – ZAR 800,000 per year
- Japan: JPY 6,000,000 – JPY 12,000,000 per year
- China: CNY 150,000 – CNY 350,000 per year
- South Korea: KRW 40,000,000 – KRW 100,000,000 per year
- Mexico: MXN 400,000 – MXN 800,000 per year
- United Arab Emirates: AED 150,000 – AED 300,000 per year
- Russia: RUB 1,500,000 – RUB 3,000,000 per year
- Italy: EUR 40,000 – EUR 80,000 per year
Wrapping Up!
Seize this opportunity to enhance both your security measures and skills, seamlessly integrating them into your daily administrative responsibilities to stay at the forefront of the industry. If you are intrigued by the motivations behind pursuing a career in cybersecurity, we recommend exploring enrollment in our Cybersecurity Master Program and following the beginner’s cybersecurity roadmap.
This comprehensive program includes courses covering CEH, PaloAlto Firewall, and CompTIA PenTest+, equipping you with the necessary certifications. Feel free to reach out with any questions or if you need assistance; we welcome your inquiries and are here to provide support. Connect with us through the comment section, and let’s embark on this cybersecurity journey together.
Happy Learning!